Accreditations
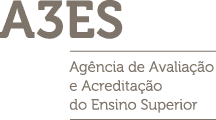
Programme Structure for 2024/2025
Curricular Courses | Credits | |
---|---|---|
1st Year | ||
Principles of Data Analysis
6.0 ECTS
|
Mandatory Courses | 6.0 |
Programming Fundamentals
6.0 ECTS
|
Mandatory Courses | 6.0 |
Applied Mathematics
6.0 ECTS
|
Mandatory Courses | 6.0 |
Work, Organizations and Technology
6.0 ECTS
|
Mandatory Courses | 6.0 |
Algorithms and Data Structures
6.0 ECTS
|
Mandatory Courses | 6.0 |
Applied Mathematics Complements
6.0 ECTS
|
Mandatory Courses | 6.0 |
Statistics and Probabilities
6.0 ECTS
|
Mandatory Courses | 6.0 |
Artificial Intelligence
6.0 ECTS
|
Mandatory Courses | 6.0 |
Project Planning and Management
6.0 ECTS
|
Mandatory Courses | 6.0 |
Public Speaking with Drama Techniques
2.0 ECTS
|
Transversal Skills | 2.0 |
Introduction to Design Thinking
2.0 ECTS
|
Transversal Skills | 2.0 |
Academic Work with Artificial Intelligence
2.0 ECTS
|
Transversal Skills | 2.0 |
2nd Year | ||
Supervised Machine Learning
6.0 ECTS
|
Mandatory Courses | 6.0 |
Database and Information Management
6.0 ECTS
|
Mandatory Courses | 6.0 |
Entrepreneurship and Innovation I
6.0 ECTS
|
Mandatory Courses | 6.0 |
Programming and Analysing Data in Excel
6.0 ECTS
|
Mandatory Courses | 6.0 |
Object Oriented Programming
6.0 ECTS
|
Mandatory Courses | 6.0 |
Autonomous Agents
6.0 ECTS
|
Mandatory Courses | 6.0 |
Unsupervised Machine Learning
6.0 ECTS
|
Mandatory Courses | 6.0 |
Entrepreneurship and Innovation II
6.0 ECTS
|
Mandatory Courses | 6.0 |
Projects in Web and Cloud Environments
6.0 ECTS
|
Mandatory Courses | 6.0 |
Analytical Information Systems
6.0 ECTS
|
Mandatory Courses | 6.0 |
3rd Year | ||
Big Data
6.0 ECTS
|
Mandatory Courses | 6.0 |
Applied Project in Artificial Intelligence I
6.0 ECTS
|
Mandatory Courses | 6.0 |
Text Mining
6.0 ECTS
|
Mandatory Courses | 6.0 |
Advanced Search Algorithms
6.0 ECTS
|
Mandatory Courses | 6.0 |
Applied Project in Artificial Intelligence II
6.0 ECTS
|
Mandatory Courses | 6.0 |
Technology, Economy and Society
6.0 ECTS
|
Mandatory Courses | 6.0 |
Principles of Data Analysis
After successfully attending the curricular unit, students should be able to:
OA1. Know the different data formats.
OA2. Know the complete data cycle.
OA3. Know how to perform exploratory data analysis using R.
OA4. Know how to model a set of data.
OA5. Implement a data analysis solution for a given problem.
CP1. Introduction to Data Analysis
CP2. Introduction to R and RStudio
CP3. Knowledge of problems in data analysis with examples
CP4. The complete cycle of data analysis
CP5. Data and data format
CP6. Data preparation
CP7. Odds; Descriptive Statistics and Exploratory Analysis
CP8. Data visualization
CP9. Modeling and different types of machine learning problems
CP10. Model evaluation methods
CP11. Reporting and publishing results
PERIODIC assessment results from: online exercises, without a minimum grade, after each class (20%); two individual tests - a mid-term test and another at the end of the semester (30%); and a group work (maximum of 3 students) in R with preparation of a report and oral presentation (50%).
Students who obtain a final grade above 9.5 are approved.
Title: Torgo Luís; Data mining with R. ISBN: 978-1-4398-1018-7
C. O'Neil, R. Schutt. 'Doing Data Science: Straight Talk from the Frontline'. O'Reilly. 2013
Authors:
Reference:
Year:
Title: Wickham, Hadley, and Garrett Grolemund. 2017. R for Data Science. O?Reilly Media.
Wilke, Claus O. 2019. Fundamentals of Data Visualization. O?Reilly Media.
P. Mathur, Machine Learning Applications Using Python: Cases Studies from Healthcare, Retail, and Finance. Apress. 2018.
I. Foster, R. Ghani, R. S. Jarmin, F. Kreuter, J. Lane, Big Data and Social Science: A Practical Guide to Methods and Tools, 1st Edition. CRC Press, Chapman & Hall. 2016
T. W. Miller, Marketing Data Science: Modeling Techniques in Predictive Analytics with R and Python?. O'Reilly. 2015
M. N. Jones, Big Data in Cognitive Science (Frontiers of Cognitive Psychology), Taylor & Francis, 2016
F. Provost. Data Science for Business: What You Need to Know about Data Mining and Data-Analytic Thinking. O'Reilly. 2013
L. M. Chen, Z. Su, B. Jiang. Mathematical Problems in Data Science: Theoretical and Practical Method
Aggarwal, C. C. 2015. Data mining: the textbook (Vol. 1). New York: Springer.
Han, J., Pei, J., & Tong, H. 2022. Data mining: concepts and techniques. Morgan Kaufmann.
P. Tattar, T. Ojeda, S. P. Murphy B. Bengfort, A. Dasgupta, Practical Data Science Cookbook, Second Edition. Packt Publishing. 2017.
Authors:
Reference:
Year:
Programming Fundamentals
By the end of this course unit, the student should be able to:
LO1: Apply fundamental programming concepts.
LO2: Create procedures and functions with parameters.
LO3: Understand the syntax of the Python programming language.
LO4: Develop programming solutions for problems of simple complexity.
LO5: Explain, execute, and debug code fragments developed in Python.
LO6: Interpret the results obtained from the execution of code developed in Python.
LO7: Develop programming projects.
S1. Introduction to Programming: Logical sequence and instructions, Input and output of data, Constants, variables, and data types, Logical, arithmetic, and relational operations, Control structures
S2. Procedures and Functions
S3. References and Parameters
S4. Integrated Development Environments
S5. Syntax of the programming language
S6. Objects and object classes
S7. Lists and Lists of Lists
S8. File Manipulation
The course unit follows a project-based assessment model due to its highly practical nature and does not include a final exam.
Students are evaluated based on the following parameters:
A1: Programming tasks validated by the instructors (10%), with a minimum grade of 9.5 out of 20 in the average of the tasks.
A2: Individual Project with theoretical-practical discussion (40%), with a minimum grade of 8.5 out of 20.
A3: Group Project with theoretical-practical discussion (50%), with a minimum grade of 8.5 out of 20.
Title: Wanda Dann, Stephen Cooper, & Randy Pausch, Learning to Program with Alice!, 2011, ISBN: 978-0132122474
João P. Martins, Programação em Python: Introdução à programação com múltiplos paradigmas, IST Press, 2015, ISBN: 9789898481474
Kenneth Reitz, Tanya Schlusser, The Hitchhiker's Guide to Python: Best Practices for Development, 1st Edition, 2016, ISBN-13: 978-1491933176, https://docs.python-guide.org/
Eric Matthes, Python Crash Course, 2Nd Edition: A Hands-On, Project-Based Introduction To Programming, No Starch Press,US, 2019, ISBN-13 : 978-1593279288
John Zelle, Python Programming: An Introduction to Computer Science, Franklin, Beedle & Associates Inc, 2016, ISBN-13 : 978-1590282755
Ernesto Costa, Programação em Python: Fundamentos e Resolução de Problemas, 2015, ISBN 978-972-722-816-4,
Authors:
Reference:
Year:
Title: David Beazley, Brian Jones, Python Cookbook: Recipes for Mastering Python 3, O'Reilly Media, 2013, ISBN-13 ? : ? 978-1449340377
Authors:
Reference:
Year:
Applied Mathematics
LG1. Review the concept of function and its properties. Types of functions and operations with functions.
LG2. Graphics of elementar functions and function transformations.
LG3. Limits, indeterminations and graphic interpretation. Continuity.
LG4. Derivatives and its applications. Graphic interpretation.
LG5. Linear approximations and higher order approximations.
LG6. Derivative of composed functions and inverse functions.
LG7. Calculations with matrices and vectors.
LG8. Calculating detrminants and applicating its proprieties.
LG9. Knowing the concept of linear transformation and representation with matrices.
LG10. Calculating eigenvalues and eigenvectors.
PC1. Function. Elementar functions, Different type of functions. Operations with functions. Logaritmic and trigonometric functions.
PC2. Limits of a function at a point, Continuity at a point. Assimptotic lines.
PC3. Derivative of a function at a point. Derivative rules. Optimization problems.
PC4. Derivative of composed functions – chain rule. Derivative of the inverse function.
PC5. Linear approximation and Taylor approximation.
PC6. Solving linear equation systems. Matrices and operations. Inverting matrices. Determinants and properties. Linear transformations.
PC7. Real vector space. Inner product. Parallelism and perpendicularity.
PC8. Eigenvalues, eigenvectores and matrix diagonalization.
Approval with classification not less than 10 points (1-20 scale) in one of the following modalities:
- Periodic assessment: 3 mini-tests (MT) on classes of 30 minutes duration (MT1: 5%, MT2: 15%, MT3: 15%) + Test on the first examination period (40%) + weekly tasks on Moodle (15%) + work done in groups of 2-3 students (10%).
The average of the classifications of mini-tests 2 and 3 ( (MT2+MT3)/2 ) must be greater or equal to 7 points.
The classification in the final test must be greater or equal to 7 points.
There is the possibility of oral assessment.
or
- Assessment by Examination (100%), in any of the examination periods.
Title: Stewart, J., Stewart, J. (2013). Cálculo, Vol I, Cengage Learning, (7a Ed.), 2013, null,
Cabral I., Perdigão, C. e Saiago, C., Cabral I., Perdigão, C. e Saiago, C. (2018). Álgebra Linear: Teoria, Exercícios Resolvidos e Exercícios Propostos com Soluções, Escolar Editora, 2018, null,
Materiais científico-pedagógicos (slides, notas de desenvolvimento, código e pseudo código, fichas de exercícios e problemas) disponibilizados pela equipa docente
Scientific-pedagogical materials (slides, lectures, code and pseudo code, exercise sheets, problems) provided by the teaching team.
Authors:
Reference:
Year:
Title: Campos Ferreira, J., Campos Ferreira, J. (2018). Introdução à Análise Matemática, Fundação Calouste Gulbenkian, 2018, null,
Goldstein, L., Goldstein, L. (2011). Matemática Aplicada a Economia. Administração e Contabilidade, (12a edição) Editora Bookman, 2011, null,
Strang, G., Strang, G., (2007) Computational Science and Engineering, Wellesley-Cambridge Press., 2007, null,
Authors:
Reference:
Year:
Work, Organizations and Technology
LO1: Know the main theories, concepts and problematics related to Work, Organizations and Technology;
LO2: Understand the main processes of the digital transition directly related to the world of work and its organizations;
LO3: Analyze the multiple social, economic and political implications of the digital transition;
LO4: Explore cases, strategies and application methods to understand the real impacts of the digital transition on professions, companies and organizations.
S1. Is work different today than in the past? S2. How has theory looked at technology?
S3. What technologies for the future?
S4. What future for work?
S5. How intelligent is artificial intelligence?
S6. Where does precarity begin?
S7. Do platform workers need employment contracts?
S8. Who is to blame when the machine goes wrong?
S9. Are digital technologies changing the relationship between unions and companies?
S10. Does teleworking make people happier?
S11. Portugal and the digital transformation?
"Periodic evaluation:
Making of an Inverted class class. Each Inverted Class represents 20% of the final mark, with a minimum mark of 8. Weekly question and answer which represents 10% of the final mark, with a minimum mark of 8. An individual assignment, spread over 3 assessment periods, with a minimum mark of 8 in each, representing 35% of the final grade. A group assignment, representing a total of 35% (10% group presentation and 25% written assignment), with a minimum mark of 8. The average grade must be equal to or greater than 9.5.
Assessment by exam (First season 1 if the student chooses, Second Season and Special Season): In-person exam (100% of the final grade)."
Title: Autor, David H., "Why Are There Still So Many Jobs? The History and Future of Workplace Automation.", 2015, Journal of Economic Perspectives, 29 (3): 3-30.
Benanav, A, Automation and the Future of Work, 2020, London: Verso
Boreham, P; Thompson, P; Parker, R; Hall, R, New Technology at Work, 2008, Londres: Routledge.
Crawford, C, The Atlas of AI. Power, Politics, and the Planetary Costs of Artificial Intelligence, 2021, Yale University Press.
Edgell, S., Gottfried, H., & Granter, E. (Eds.). (2015). The Sage Handbook of the sociology of work and employment.
Grunwald, A. (2018). Technology Assessment in Practice and Theory. London: Routledge.
Huws, U. (2019) Labour in Contemporary Capitalism, London, Palgrave.
OIT (2020), As plataformas digitais e o futuro do trabalho
Agrawal A, Gans J, Goldfarb A (2018), Prediction Machines, Boston, Massachusetts, Harvard Business Review Press.
Autor D (2022), The labour market impacts of technological change, Working Paper 30074, NBER Working Paper Series.
Authors:
Reference:
Year:
Title: Berg J, Furrer M, Harmon E, Rani U, Silberman M (2020), As plataformas digitais e o futuro do trabalho, Geneva, International Labour Office.
Braun J, Archer M, Reichberg G, Sorondo M (2021), Robotics, AI and Humanity, Cham, Springer.
Degryse, Cristophe (2016), Digitalisation of the Economy and its Impact on Labour Markets, WP 2016.2, ETUI
ILO (2018), The economics of artificial intelligence: Implications for the future of work, Geneva, International Labour Office.
ILO (2019) Work for a Brighter Future – Global Commission on the Future of Work. Report. Geneva, International Labour Office.
Lane M, Saint-Martin A (2021), The impact of Artificial Intelligence on the labour market: What do we know so far?, OECD.
OECD (2019b), How’s Life in the Digital Age?, OECD Publishing, Paris.
Valenduc, Gérard & Vendramin, Patricia (2019), The mirage of the end of work, FB 6/2019, ETUI
WEF (2023), Future of Jobs Report 2023, Geneva, World Economic Forum.
Zuboff S (2019), The Age of Surveillance Capitalism, PublicAffairs.
Authors:
Reference:
Year:
Algorithms and Data Structures
At the end of the course, students should be able to:
LO1: Create and Manipulate Data Structures
LO2: Apply the most appropriate sorting and search algorithms for a specific problem
LO3: Analyze the complexity and performance of an algorithm
LO4. Identify, implement, and analyze the most appropriate data structures and algorithms for a certain problem
S1. The Union-Find data structure
S2. Algorithm analysis
S3: Data structures: stacks, queues, lists, bags
S4: Elementary sorting: selectionsort, insertionsort, shellsort
S5: Advanced sorting: mergesort, quicksort, heapsort
S6. Complexity of sorting problems
S7: Priority Queues
S8. Elementary symbol tables
S9. Binary search trees
S10. Balanced search trees
S11. Hash tables
Season 1: Periodic Assessment or Final Exam
Periodic Assessment:
-2 Tests (90%), with a theoretical and practical component. Minimum final average of 9.5, distributed as follows: (45%) Test 1 with a minimum score of 7.5 and (45%) Test 2 with a minimum score of 7.5
-(10%) Application and demonstration of knowledge tasks
Final Exam:
- (100%) Final Exam with a theoretical and practical component
Students have access to the Exam assessment in Season 1 if they choose it at the beginning of the semester or if they fail the Periodic Assessment.
Season 2: Final Exam
- (100%) Final Exam with a theoretical and practical component
Special Season: Final Exam
- (100%) Final Exam with a theoretical and practical component
Title: Para as licenciaturas Python: Python - Algoritmia e Programação Web, FCA,
Para as licenciaturas Java: Estruturas de Dados e Algoritmos em Java, FCA
Introduction to Algorithms, 3rd edition, MIT Press,
Algorithms, 4th edition, Addison-Wesley, 2012
Authors:
Reference:
Year:
Applied Mathematics Complements
LG1 Dominate the concepts of sequence and numerical series
LG2 Calculate limits of sequences and, relative to a series, find out the existence of sum
LG3 Understand the generalization of the concept of series to functional series and obtain the convergence domain
LG4 Understand the definition of integral as the limit of Riemann sums
LG5 Calculate primitives and apply them to determine the value of integrals
LG6 Apply integrals to calculate areas, lengths and mean values
LG7 Solve 1st order linear ordinary differential equations (ODEs) by separating variables
LG8 Calculate partial derivatives and directional derivative
LG9 Interpret the gradient vector as the direction of maximal increase of a function
LG10 Decide about the existence of a tangent plane
LG11 Obtain the 1st order Taylor development and, explore numerically in higher order
LG12 Obtain unconstrained and constrained extrema(otimization)
LG13 Articulate the various approaches to content, graphical, numerical and algebraic
PC1 Sequences. Monotony. Bounded sequences. Geometric progression
PC2 Convergence of sequences
PC3 Numerical series, partial sums and sum
PC4 Convergence criteria of series of non-negative terms
PC5 Simple and absolute convergence of alternating series. Leibniz's criterion
PC6 Power series and domain of convergence
PC7 Riemann definite integral. Fundamental theorem of calculus and antiderivatives
PC8 Integration by parts and change of variables. Decomposition into simple fractions
PC9 Applications of integral (area, length, mean value)
PC10 Improper integral and convergence
PC11 First order linear ODE
PC12 Multivariable real functions. Level curves. Limits and continuity
PC13 Partial derivatives at a point and gradient vector. Linear approximation, tangent plane and differentiability
PC14 Directional derivative. Chain rule. Taylor's polynomials and series
PC15 Quadratic forms and otimization problems
Approval with classification >=10 points (1-20 scale) in one of the following modalities:
-Continuous assessment: Test 1 (10%) + Test 2 (20%) + practical work in Python (10%) + autonomous work (10%) + Final Test (40%). The average of the 2 tests and the classification on the final test must be >=7 points (1-20 scale). In case of big differences in the classifications on tests and final test, an oral assessment might be necessary.
-Assessment by Exam (100%), in any of the exam periods
Title: [1] Stewart, J. (2013). Cálculo, Vol I, Cengage Learning, (7ª Ed.)
[2] Campos Ferreira, J. (2018). Introdução à Análise Matemática, Fundação Calouste Gulbenkian
[3] Lipsman, R.L., Rosenberg, J.M. (2018) Multivariable Calculus with MATLAB, Springer
[4] Hanselman, D., Littlefield, B. and MathWorks Inc. (1997) The Student Edition of MATLAB, 5th Version, Prentice-Hall
Authors:
Reference:
Year:
Statistics and Probabilities
LG1 - Know and use the main concepts of descriptive statistics, choose appropriate measures and graphical representations to describe data
LG2 - Apply basic concepts of probability theory, namely compute conditional probabilities, and check for independence of events
LG3 - Work with discrete and continuous random variables.
LG4 - Work and understand the uniform, Bernoulli, binomial, Poisson, Gaussian distribution, as well as Chi-Square, t and F distributions
LG5 - Perform point parameter estimation and distinguish parameters from estimators
LG6 - Build and interpret confidence intervals for parameter estimates
LG7 - Understand the fundamentals of hypothesis testing
LG8 - Get familiar with some software (such Python or R)
Syllabus contents (SC):
SC1 - Descriptive statistics: Types of variables. Frequency tables and graphical representations. Central tendency measures. Measures of spread and shape.
SC2 - Concepts of probability theory: definitions, axioms, conditional probability, total probability theorem and Bayes's formula
SC3 - Univariate and bivariate random variables: probability and density functions, distribution function, mean, variance, standard deviation, covariance and correlation.
SC4 - Discrete and Continuous distributions: Uniform discrete and continuous, Bernoulli, binomial, binomial negative, Poisson, Gaussian, Exponential Chi-Square, t and F distributions.
SC 5 - Sampling: basic concepts. Most used sample distributions
SC6 - Point estimation and confidence intervals
SC7 - Hypothesis testing: types of errors, significance level and p-value
Approval with a mark of not less than 10 in one of the following methods:
- Periodic Assessment: 2 mini-tests (MT) taken in class (15% each) + Final test taken on the date of the first exam (40%) + autonomous work (10%) + group project (20%),
The average of the mini-tests ( (MT1+MT2)/2 ) has a minimum mark of 7.0.
The final test has a minimum mark of 7.0.
or
- Assessment by Exam (100%).
Title: E. Reis, P. Melo, R. Andrade & T. Calapez, Estatística Aplicada (Vol. 1) - 6ª ed, 2015, Reis, E., P. Melo, R. Andrade & T. Calapez (2015) Estatística Aplicada (Vol. 1), 6ª ed., Lisboa: Sílabo. ISBN: 978-989-561-186-7, ·
Reis, E., P. Melo, R. Andrade & T. Calapez (2016) Estatística Aplicada (Vol. 2), 5ª ed., Lisboa: Sílabo. ISBN: 978-972-618-986-2
Afonso, A. & Nunes, C. (2019). Probabilidades e Estatística. Aplicações e Soluções em SPSS. Versão revista e aumentada. Universidade de Évora. ISBN: 978-972-778-123-2
Ferreira, P.M., Estatística e Probabilidade (Licenciatura em Matemática), 2012, Ferreira, P. M. (2012). Estatística e Probabilidade (Licenciatura em Matemática). Instituto Federal de Educação, Ciência e Tecnologia do Ceará – IFCE III, Universidade Aberta do Brasil – UAB.IV. ISBN: 978-85-63953-99-5,
Farias, A. (2010). Probabilidade e Estatística. (V. único). Fundação CECIERJ. ISBN: 978-85-7648-500-1
Authors:
Reference:
Year:
Title: Haslwanter, T. (2016). An Introduction to Statistics with Python: With Applications in the Life Sciences. Springer. ISBN: 978-3-319-28316-6
Authors:
Reference:
Year:
Artificial Intelligence
Upon completion of the course, students should:
LO1: Recognize the advantages and challenges of using Artificial Intelligence (AI) techniques and approaches, demonstrating critical awareness of informed and uninformed search methods.
LO2: Select and justify the most appropriate technological approaches and algorithms, including search methods, representation, and reasoning logics.
LO3: Apply the concepts and techniques discussed in the design and development of AI-based systems, as well as in the modeling of examples based on real scenarios.
LO4: Develop, implement, and evaluate solutions involving predicate logic and logic programming.
LO5: Understand the fundamentals of genetic algorithms, being able to implement and adapt them to solve specific problems.
LO6: Work autonomously and in groups to develop projects that apply the acquired knowledge, demonstrating the ability to adapt and solve complex problems in the AI field.
S1: Fundamental notions of AI with emphasis on the search-based approach.
S2: Search algorithms: depth first and breadth first, A*, greedy BFS, Dijkstra.
S3: Fundamental notions relating to knowledge, representation and the architecture of knowledge-based systems.
S4: First-order predicate logic: representation and deduction.
S5: Declarative knowledge represented in Logic Programming.
S6: Genetic algorithms.
The periodic assessment throughout the semester consists of 3 assessment blocks (BA), and each BA consists of one or more assessment moments. It is distributed as follows:
- BA1: 4 mini-assignments [7.5% each mini-assignment * 4 = 30%]
- BA2: 2 mini-tests [20% each mini-test * 2 = 40%]
- BA3: 1 project on Artificial Intelligence [30%]
Assessment by exam:
- 1st Epoch [100%]
- 2nd Epoch [100%]
All periodic assessment blocks (BA1, BA2 and BA3) have a minimum grade of 8.5. In any BA, an individual oral discussion may be required.
Assessment by exam consists of a written exam that covers all the knowledge provided in the syllabus of the course, and has a weighting of 100%.
Attendance at classes is not mandatory.
Title: Bishara, M. H. A., & Bishara, M. H. A. (2019). Search algorithms types: Breadth and depth first search algorithm
Brachman, R., & Levesque, H. (2004). Knowledge representation and reasoning. Morgan Kaufmann
Clocksin, W. F., & Mellish, C. S. (2003). Programming in Prolog. Springer Berlin Heidelberg.
Russell, S. & Norvig, P. (2010). Artificial Intelligence: A Modern Approach (3rd ed.). Prentice Hall.
S., V. C. S., & S., A. H. (2014). Artificial intelligence and machine learning (1.a ed.). PHI Learning.
Authors:
Reference:
Year:
Project Planning and Management
The objective of the UC is to develop a technological project in line with the scope of the Course. Contact will be established with project planning considering the main phases: Requirements analysis, development, partial tests and final tests and changes. Contact with laboratory equipment and tools is one of the goals for designing a software, hardware or both project.
ProgramI. Introduction to technological innovation along the lines of Europe
II. Planning a technological project and its phases
III. Essential aspects for the development of a project
IV. Definition of material resources
V. Budget of a project
VI. Partial and joint Test Plan
VII. Presentation of a technological project
VIII. Technological project demonstration
IX. Preparation of Technical Report
Periodic grading system:
- Group project: first presentation: 30%; second presentation and exibithion: 40%; final report: 30%. The presentations, demonstrations and defence are in group.
Title: Lester A. / 7th edition, Elsevier Science & Technology., Project Management Planning and Control, 2017, ·, ·
Tugrul U. Daim, Melinda Pizarro, e outros / Spinger, Planning and Roadmapping Technological Innovations: Cases and Tools (Innovation, Technology, and Knowledge Management), 2014, ·, ·
Authors:
Reference:
Year:
Public Speaking with Drama Techniques
Learning Outcomes:
LO1. Develop oral communication skills
LO2. Improve body expression
LO3. Master the art of using the vocal apparatus
LO4. Learn performance techniques
Compatibility with the Teaching Method:
The course combines theory and practice, providing students with an immersive experience in the world of public performances with theatrical techniques. The teaching method is interactive and participatory, encouraging students to put into practice the concepts learned through individual and group exercises.
The knowledge acquired involves both theatrical theory and specific oral communication techniques. Participants will learn about the fundamentals of vocal expression, character interpretation and improvisation, adapting these skills to the context of public presentations
S1 - Preparation for presentation (3 hours)
S2 - Non verbal communication (3 hours)
S3 - Introduction to using the vocal apparatus (3 hours)
S4 - Introduction to the term Performance (3 hours)
Modality of continuous assessment:
Practical Presentations (50%): Participants will be assessed based on their public presentations during the course. Criteria such as clarity of communication, vocal and body expression, use of theatrical techniques and performance will be considered. Presentations may be individual or group presentations, depending on the activities proposed.
Exercises and Written Assignments (50%): In addition to the practical presentations, participants may be asked to complete exercises and written assignments related to the content covered in each module. These may include reflections on learned techniques, analysis of case studies, answers to theoretical questions or even the creation of presentation scripts. These activities will help to assess participants' conceptual understanding.
To conclude the curricular unit in the modality of continuous assessment the student must be present in 75% of the classes.
Although not recommended, students may opt for final assessment by written and oral examination (100%).
Title: -
Authors:
Reference:
Year:
Title: -
-
Authors:
Reference:
Year:
Introduction to Design Thinking
LO1. Acquiring knowledge about the fundamentals and stages of the Design Thinking process
LO2. Develop skills such as critical thinking, collaboration, empathy and creativity.
LO3. To apply Design Thinking in problem solving in several areas, promoting innovation and continuous improvement.
S1. Introduction to Design Thinking and Stage 1: Empathy (3h)
S2. Steps 2 and 3: Problem Definition and Ideation (3h)
S3. Step 4: Prototyping (3h)
S4. Step 5: Testing and application of Design Thinking in different areas (3h)
Modality of continuous assessment:
Class participation (20%): evaluates students' presence, involvement and contribution in class discussions and activities.
Individual work (40%): students will develop an individual project applying Design Thinking to solve a specific problem. They will be evaluated on the application of the stages of Design Thinking, quality of the proposed solutions, and creativity.
Group work (40%): students will form groups to develop a joint project, applying Design Thinking to solve a real challenge. Evaluation will be based on the application of the steps of Design Thinking, quality of the solutions and collaboration among group members.
To conclude the curricular unit in the modality of continuous assessment the student must be present in 75% of the classes.
Although not recommended, students may opt for final assessment by written and oral examination (100%).
Title: -
-
-
-
Authors:
Reference:
Year:
Title: -
-
-
-
Authors:
Reference:
Year:
Academic Work with Artificial Intelligence
"LO1. Knowledge about the structure, language, ethical and normative procedures for the elaboration of academic texts.
LO2. Skills to use generative algorithms to assist the elaboration of academic work.
LO3. Skills in analysing and scrutinising the independence, relevance and reliability of AI generated data.
LO4. Overall abilities to recognise the ethical and civic implications underlying the access, sharing and use of AI tools in an academic context."
"S1. Introduction to Academic Writing and generative algorithms (3h)
S2. Procedures for planning and constructing argumentative texts with the aid of AI (3h)
S3. Critical analysis of texts produced: identification and referencing of data sources and analysis of their relevance in the ligth of the objectives of the academic work (3h)
S4. Opportunities and risks of AI use: good practice guide for accessing, sharing and using AI tools in an academic context (3h)"
"Modality of continuous assessment:
Class participation: Class participation: assesses students' attendance, involvement and individual contributions to class discussions and activities (20%).
Group work will require students to form groups to revise and edit academic texts between themselves, using generative algorithms. Assessment will be based on the quality of the revisions, edits and feedback provided (40%).
Individual report: with an in-depth reflection on the civic and ethical questions posed by the use of AI tools as an aid to academic writing (40%).
There is a required minimum of 7 values in each component that is graded.
To conclude the curricular unit in the modality of continuous assessment the student must be present in, at least, 75% of the classes.
Although not recommended, students may opt for final assessment by written and oral examination (100%).
In addition to the practical presentations, students will be asked to carry out exercises and written tasks related to the content covered. These may include: reflecting on techniques learnt, analysing case studies, answering theoretical questions or even creating presentation scripts. These activities will help to assess conceptual understanding of the content taught.
Title: -
-
-
-
Authors:
Reference:
Year:
Title: -
Authors:
Reference:
Year:
Supervised Machine Learning
LO1. Know the history of machine learning; know and understand the different types of machine learning: concepts, foundations and applications.
LO2. Know the concepts that enable Exploratory Data Analysis (EDA) to be carried out, as well as understanding its importance in problem-solving and decision-making.
LO3. Learn Data Wrangling mechanisms - preparing data for input to a supervised algorithm.
LO4. Know how to use continuous and categorical variables; distinguish between classification and regression
LO5. Know and analyze the results by applying performance evaluation metrics
LO6. Understand supervised algorithms: decision trees, linear and logistic regression, SVMs, Naive-Bayes and k-NN.
LO7. Understand ensemble algorithms: bagging and boosting
LO8. Know and understand the workings of Artificial Neural Networks (ANN)
LO9. Know and understand hyperparameter optimization
S1. Introduction to Machine Learning: The history, foundations and basic concepts
S2. Exploratory Data Analysis (EDA): Data Wrangling and Data Visualization
S3. Classification and Regression; Continuous and categorical / discrete variables; performance evaluation metrics
S4. Supervised Learning: SVM, Decision Trees, Linear and Logistic Regression, Naive-Bayes and k-NN.
S5. Bagging and Boosting in supervised algorithms
S6. Artificial Neural Networks
S7. Hyperparameter optimization
As this course is of a very practical and applied nature, it follows the 100% project-based assessment model, which is why there is no final exam. Assessment takes place throughout the semester and consists of 3 assessment blocks (AB), each AB consisting of one or more assessment moments. It is distributed as follows:
- AB1: 1st tutorial + 1st mini-test [20% for the 1st tutorial + 10% for the 1st mini-test = 30%]
- AB2: 2nd tutorial + 2nd mini-test [20% for the 2nd tutorial + 10% for the 2nd mini-test = 30%]
- AB3: 1 final project [40%]
The tutorials consist of individual oral discussions to assess the students' performance in the projects proposed for the tutorial.
The mini-tests make it possible to assess the theoretical knowledge applied to each of the projects also assessed during the tutorial.
The final project consists of developing a practical piece of work that brings together the knowledge and skills acquired throughout the semester, in which external organizations/companies may participate in the proposed challenge.
The 1st Season and 2nd Season can be used for assessment.
Attendance at classes is not mandatory.
Title: Witten, I. H., Frank, E., Hall, M. A., & Pal, C. J. (2016). Data Mining: Practical machine learning tools and techniques. Morgan Kaufmann.
Sharda, R., Delen, D., Turban, E., Aronson, J., & Liang, T. P. (2014). Businesss Intelligence and Analytics: Systems for Decision Support. Prentice Hall.
VanderPlas, J. (2016). Python Data Science Handbook. O’Reilly Media.
Authors:
Reference:
Year:
Title: Foster Provost, Tom Fawcett (2013) Data Science for Business. What you need to know about data mining and data-analytic thinking, 1st edition. O'Reilly.
Ller, A. & Guido, S. (2017). Introduction To Machine Learning with Python: A Guide for Data Scientists. Sebastopol, CA: O'Reilly Media, Inc.
Avila, J. (2017). Scikit-Learn Cookbook - Second Edition. Birmingham: Packt Publishing.
Mueller, J. P. (2019). Python for Data Science for Dummies, 2nd Edition (2.a ed.). John Wiley & Sons.
McKinney, W. (2022). Python for Data Analysis: Data Wrangling with Pandas, NumPy, and Jupyter (3.a ed.). O’Reilly Media.
Authors:
Reference:
Year:
Database and Information Management
LO1 Know the basic principles of Information Systems and their role in organizations
LO2 Know the fundamental concepts of Information Systems Analysis and develop semantic (conceptual) models for systems described in text, through practical application of the UML language, and understand the conversion of such conceptual models into relational database models (RDBs)
LO3 Know how to model and design a Relational DB (RDB), with the Relational Model
LO4 Know the normal forms and relational algebra and understand the normalization of an existing RDB based on performance metrics
LO5 Know how to create and modify the physical structure of a RDB using SQL
LO6 Know how to use, at an elementary level, the administration tools associated with a Database Management System (DBMS)
LO7 Develop self-learning, peer review, teamwork, oral and written expression
S1 Introduction to Information Systems and its role in organizations
S2 Introduction to Information Systems Analysis with UML language: requirements analysis, data models, schemas and UML diagrams
S3 Database Design. Relational Model: relationships, attributes, primary keys, foreign keys, integrity rules, optimizations and indexes
S4 Normalization. Redundancy and inconsistency of data. Normal forms
S5 SQL Language - Table variables, set operators, simple queries, subqueries, operators (SELECT, Insert, delete, update), views, indexes, triggers, stored procedures and transactions
S6 Introduction to Database Management Systems administration, DBMS
Periodical Assessment:
- 1 test to be done in the middle of the semester (30%)
- 1 test to be taken in the 1st season of exams (30%)
- 1 modelling and implementation project (40%)
Both tests have a minimum grade of 8 values and the project is mandatory for approval.
Assessment by exam:
-1 Written exam weighted at 100%
The minimum grade for approval in this course is 10 values.
Title: Ramos, P, Desenhar Bases de Dados com UML, Conceitos e Exercícios Resolvidos, Editora Sílabo, 2ª Edição, 2007
Elmasri Ramez, Navathe Shamkant, "Fundamentals Of Database Systems", 7th Edition, Pearson, 2016
Damas, L., SQL - Structured Query Language, FCA Editora de Informática, 3ª Edição,2017
Authors:
Reference:
Year:
Title: Nunes, O´Neill, Fundamentos de UML, FCA Editora de Informática, 3ª Edição, 2004
C. J. Date, "SQL and Relational Theory: How to Write Accurate SQL Code", 3rd Edition, O'Reilly Media, 2011
Authors:
Reference:
Year:
Entrepreneurship and Innovation I
At the end of the learning unit, the student must be able to: LG.1. Understand entrepreneurship; LG.2. Create new innovative ideas, using ideation techniques and design thinking; LG.3. Create value propositions, business models, and business plans; LG.5. Develop, test and demonstrate technology-based products, processes and services; LG.6. Analyse business scalability; LG.7. Prepare internationalization and commercialization plans; LG.8. Search and analyse funding sources
ProgramI. Introduction to Entrepreneurship;
II. Generation and discussion of business ideas;
III. Value Proposition Design;
IV. Business Ideas Communication;
V. Business Models Creation;
VI. Business Plans Generation;
VII. Minimum viable product (products, processes and services) test and evaluation;
VIII. Scalability analysis;
IX. Internationalization and commercialization;
X. Funding sources
Periodic grading system: - Group project: first presentation: 30%; second presentation: 30%; final report: 40%.
Title: A. Osterwalder, Y. Pigneur / John Wiley & Sons, Value Proposition Design: How to Create Products and Services Customers Want, 2014, ·, ·
A. Osterwalder, Y. Pigneur / John Wiley & Sons, Business Model Generation: A Handbook for Visionaries, Game Changers, and Challengers., 2010, ·, ·
P. Burns / Palgrave Macmillan, Entrepreneurship and Small Business, 2016, ·, ·
S. Mariotti, C. Glackin / Global Edition. Pearson; Dorf. R., Byers, T. Nelson, A. (2014). Technology Ventures: From Idea to Enterprise. McGraw-Hill Education, Entrepreneurship: Starting and Operating A Small Business, 2015, ·, ·
Authors:
Reference:
Year:
Programming and Analysing Data in Excel
With this curricular unit, the student should be able to:
LO1: Describe the variables and the formulas needed to construct a calculation model;
LO2: Use the basic features of a spreadsheet;
LO3: Use functions for building models in a spreadsheet;
LO4: Construct advanced models applied to management in a spreadsheet;
LO5: Compute automatic procedures with the use of VBA.
S1: Introduction to spreadsheets
S2: Model construction
S3: Information extraction
S4: Introduction to Visual Basic for Applications
Periodic Assessment:
- Group work (50%) - A project made by a group of students.
- Individual Test (50%) - Written test, scheduled to be taken in person, via the e-learning platform. This test will have a minimum score of 8.
Final exam:
Individual written exam, without consultation, encompassing the entire syllabus. This is for those who have not made both predicted assessments or have not obtained an average greater than or equal to 10 (out of 20), in the set of the two assessments.
Title: Winston, Wayne L. (2019). Microsoft Excel 2019: Data Analysis and Business Modeling, 6th Edition, Pearson Education, USA., ISBN 978-150-930-588-9.
Martins, António (2017). Excel Aplicado à Gestão, 4ª Edição, Edições Sílabo, Lisboa, ISBN 978-972-618-876-6.
Dias Curto, José, & Vieira, Duarte, Excel para Economia e Gestão, 2023, 5ª Ed., Edições Sílabo, Lisboa, ISBN 978-989-561-290-1.,
Lomonaco, Leonardo, A Comprehensive Guide to Become an Expert on Excel 2023 With All-in-One Approach, 2023, 1st. Ed., Leonardo Lomonaco, Author edition, ISBN 979-821-534-773-7.,
Authors:
Reference:
Year:
Title: Barlow, John F. (2005). Excel Models for Business and Operations Management, 2nd Edition, John Wiley & Sons, USA, ISBN 978-047-001-509-4.
Authors:
Reference:
Year:
Object Oriented Programming
LO1 Structure the students' logical thinking in order to solve programming problems.
LO2 Empower students with the ability to perceive the object-oriented programming paradigm.
LO3 Use an object-oriented programming language and the necessary tools, to design, develop, test and debug small applications.
LO4 Understand and apply the concepts of abstraction, encapsulation, inheritance and polymorphism.
LO5 Know how to use the fundamental data structures of a library (stacks, queues, trees, scatter tables).
LO6 Apply error control mechanisms.
LO7 Explain the usefulness of using software design patterns and demonstrate their use in simple cases.
LO8 Develop creativity, technological innovation and critical thinking.
LO9 Develop self-learning, peer review, teamwork, and oral expression.
S1 Classes and Objects
S2 Inheritance and polymorphism
S3 Abstract classes
S4 Interfaces and comparators
S5 Collections: lists, sets, maps
S6 Anonymous classes and lambdas
S7 Reading and writing files
S8 Exceptions and error handling
S9 Genericity and design patterns
S10 JUnit Tests and Documentation
CU with Periodic Assessment, not including a Final Exam:
8 individual assignments (10%), min grade of 9.5
Group project, with oral discussion (45%), min grade of 9.5
2 Mini-tests (45%), minimum grade of 9.5
If failing in the first period (< 10 out of 20), the student can retake the 1st and/or 2nd mini-tests (can also retake if scoring below the minimum grade in either or both) - accounting for 55% of the grade, with passing the Project or completing an individual project being mandatory - 45%
Title: F. Mário Martins, "Java 8 POO + Construções Funcionais", FCA - Editora de Informática, 2017. ISBN: 978-972-722-838-6
Y. Daniel Liang, "Introduction to Java Programming: Comprehensive Version" 10th Ed. Prentice-Hall / Pearson, 2015.
Recursos Java http://java.sun.com
Authors:
Reference:
Year:
Title: Ken Arnold, James Gosling e David Holmes, "The JavaTM Programming Language", 3ª edição, Addison-Wesley, 2000.
ISBN: 0-201-70433-1
Bruce Eckel, "Thinking in Java", 3ª edição, Prentice Hall, 2002. ISBN: 0-13-100287-2
Gamma, Helm, Johnson & Vlissides (1994). Design Patterns. Addison-Wesley. ISBN 0-201-63361-2.
Authors:
Reference:
Year:
Autonomous Agents
It aims to introduce fundamental concepts and practical knowledge to the use and development of software agents. After completing the UC, students must:
(LO1) Be aware of the advantages and challenges of using and developing agents immersed in a society of human actors and other artificial agents;
(LO2) Know how to identify the requirements regarding the agents to be developed, in terms of agent roles and communication;
(LO3) Choose and implement the most appropriate approaches to autonomous behavior control;
(LO4) Master the agent communication language and the content language used in this communication.
(LO5) Know the mechanisms necessary for coordinating agents in society, taking into account users’ objectives;
(LO6) Identify needs that can be satisfied through the use of software agents and critically analyze the benefits and disadvantages expected when using this technology.
(P1) Agent-oriented programming. Agent-based simulation versus multi-agent systems. Tools for developing multi-agent systems and agent-based simulation.
(P2) Agents and environments. Basic types of agents: deductive; reactives; deliberative; emotional, adaptive. Agents with reinforcement learning; agents with incremental data mining; hybrid agents.
(P3) Multi-agent systems. Coordination, stigmeria , self-organization, emergence and communication. Speech acts – ACL and FIPA.
(P4) Multi-agent decision making . Game theory. Utilities and preferences. Dominant strategies. Nash equilibrium . Pareto optimal . Uncertainty. Cooperative games. Negotiating agents. Auction agents. Mechanism design. Argumentative agents.
(P5) Introduction to agent-based simulation. Agent-based modeling and simulation (ABMS). Agent-based models and complex adaptive systems.
(P6) Ethical issues in the construction of agents.
Periodic assessment results from the following components:
- A midterm test (20% of the final grade) and another at the end of the semester (20% of the final grade);
- Group work (maximum 3 students) in which the group will develop the prototype of a multi-agent system with the preparation of a report involving 3 deliverables to be submiteed along the semester (30% of the final grade, 10% each deriverable) and an oral presentation demonstrating the functioning of the developed software (30% of the final grade).
Alternatively, students may choose to be assessed in a final exam (100% of the final grade)
Students who obtain a final grade above 9.5 are approved.
Title: Wooldridge, M. (2009). An Introduction to MultiAgent Systems - 2nd Edition. In ACM SIGACT News (Vol. 41, Issue 1)
Authors:
Reference:
Year:
Title: Vlassis, N. (2007). A Concise Introduction to Multiagent Systems and Distributed Artificial Intelligence. In Synthesis Lectures on Artificial Intelligence and Machine Learning (Vol. 1, Issue 1). https://doi.org/10.2200/s00091ed1v01y200705aim002
Leyton-brown, K. (2009). Multiagent Systems: Algorithmic, Game-Theoretic, and Logical Foundations.
Gerhard, W. (n.d.). Multiagent systems (2nd ed). MIT press.
Authors:
Reference:
Year:
Unsupervised Machine Learning
LO1. Understand the main methods of non-supervised learning.
LO2: Evaluate, validate, and interpret the results of non-supervised models.
LO3: Develop knowledge discovery projects from data using non-supervised learning models.
LO4: Acknowledge, through the approach of various problem contexts (e.g., customer segmentation) in which unsupervised learning can effectively provide solutions relevant to these problems.
LO5. Understand the theoretical and practical foundations of reinforced learning.
LO6. Implement and test reinforced learning algorithms in simulated environments to understand the dynamics between actions and consequent rewards.
LO7. Evaluate and optimize the performance of reinforced learning models using appropriate metrics.
LO8. Learn and apply unsupervised and reinforced algorithms in practical case studies.
SY1: Introduction to unsupervised learning: fundamental concepts, types of algorithms and practical applications.
SY2: Dimensionality reduction and data visualization: Principal Component Analysis (PCA), t-SNE and UMAP for dimensionality reduction and visual interpretation.
SY3: Clustering and segmentation techniques: exploration of algorithms such as K-Means, DBSCAN, Expectation-Maximization (EM), hierarchical clustering.
SY4: Outlier analysis and detection using unsupervised techniques: KNN, LOF, iForest.
SY5: Association rules and the Apriori algorithm.
SY6: Self-Organizing Maps (SOMs): application of self-organizing maps for visualization and analysis of complex patterns in large volumes of data.
SY7: Reinforcement learning techniques: Q-Learning, SARSA. Introduction to concepts and practical implementation.
SY8: Exploration vs. exploitation in reinforcement learning: strategies for balancing decision-making.
Being a course with a very practical and applied nature, it follows the 100% project-based assessment model, where there is no final exam. Assessment takes place throughout the semester and consists of 3 assessment blocks (AB), each consisting of one or more assessment moments. The distribution is as follows:
- AB1: 1st tutorial + 1st mini-test [20% 1st tutorial + 10% 1st mini-test = 30%]
- AB2: 2nd tutorial + 2nd mini-test [20% 2nd tutorial + 10% 2nd mini-test = 30%]
- AB3: 1 final project [40%]
The tutorials consist of individual oral discussions to assess the students' performance in the projects proposed for the tutorial.
The mini-tests evaluate the theoretical knowledge applied to each of the projects also assessed during the tutorial.
The final project consists of developing a practical piece of work that brings together the knowledge and skills acquired throughout the semester, in which external organizations/companies may participate in the proposed challenge.
The 1st Epoch and 2nd Epoch can be used for all the assessments.
Attendance at classes is not mandatory.
Title: Berry, M. W., Mohamed, A., & Yap, B. W. (Eds.). (2019). Supervised and unsupervised learning for data science. Springer Nature.
Vidal, R., Ma, Y., & Sastry, S. S. (2016). Generalized principal component analysis (Vol. 5). New York: Springer.
Reddy, C. K. (2018). Data Clustering: Algorithms and Applications. Chapman and Hall/CRC.
Szepesvari, C. (2010). Algorithms for reinforcement learning (R. Brachman & T. Dietterich, Eds.; 1.a ed.). Morgan & Claypool.
Authors:
Reference:
Year:
Title: Kassambara, A. (2017). Practical guide to cluster analysis in R: Unsupervised machine learning (Vol. 1). Sthda.
Verdhan, V. (2020). Models and Algorithms for Unlabelled Data. Springer.
Contreras, P., & Murtagh, F. (2015). Hierarchical clustering. In Handbook of cluster analysis (pp. 124-145). Chapman and Hall/CRC.
Sutton, R. S., & Barto, A. G. (2018). Reinforcement Learning, second edition: An Introduction (2.a ed.). MIT Press.
Authors:
Reference:
Year:
Entrepreneurship and Innovation II
At the end of this UC, the student should be able to:
LG.1. Present the image of the product/service in a website
OA.2. Present the image of the product/service in social networks
OA.3. Describe functionalities of the product/service
OA.4. Describe phases of the development plan
OA.5. Develop a prototype
OA.6. Test the prototype in laboratory
OA.7. Correct the product/service according to tests
OA.8. Optimize the product/service considering economic, social, and environmental aspects
OA.9. Adjust the business plan after development and tests, including commercialization and image
OA.10. Define product/service management and maintenance plan
I. Development of the product/service image
II. Functionalities of the product/service
III. Development plan
IV. Development of the product/service (web/mobile or other)
V. Revision of the business plan
VI. Management and maintenance of the product/service
VII. Certification plan
VIII. Intellectual property, patents, and support documentation
IX. Main aspects for the creation of a startup - juridical, account, registry, contracts, social capital, obligations, taxes
Periodic grading system:
- Group project: first presentation: 30%; second presentation: 30%; final report: 40%. The presentations, demonstrations and Defence are in group.
Title: ·
A. Osterwalder, Y. Pigneur / John Wiley & Sons, Value Proposition Design: How to Create Products and Services Customers Want, 2014, ·, ·
A. Osterwalder, Y. Pigneur / John Wiley & Sons, Business Model Generation: A Handbook for Visionaries, Game Changers, and Challengers, 2010, ·, ·
P. Burns / Palgrave Macmillan, Entrepreneurship and Small Business, 2016, ·, ·
R. Dorf, T. Byers, A. Nelson / McGraw-Hill Education, Technology Ventures: From Idea to Enterprise., 2014, ·, ·
S. Mariotti, C. Glackin / Global Edition. Pearson, Entrepreneurship: Starting and Operating A Small Business, 2015, ·, ·
Authors:
Reference:
Year:
Projects in Web and Cloud Environments
LO1: To understand Web technologies, programming languages for Web, and usual architectures
LO2: Identify and explain different types of cloud architecture and their key features;
LO3: Identify and use the key technologies enabling cloud computing;
LO4: Propose appropriate cloud architectures for a particular application;
SY1: Standard W3C and Web programming
SY2: Client-Server architectures
SY3: Model View Controler (MVC)
SY4: Cloud Principles and Business Drivers;
SY5: Pre-Cloud technology, Virtualisation, Hypervisors, Xen, Virtual Clusters;
SY6: XaaS, Public, Private, Hybrid Clouds, Examples;
SY7: Basics on the development of Cloud applications;
This Unit is accomplished through 2 projects that worth 50% each:
(1) Development of a Web Application
(2) Development of a cloud-based project
In the first evaluation period, the project is developed in groups of students with an individual discussion (that accounts for 50% of the grade in each project). As for the second and extra evaluation period, the projects are developed individuallyl.
Title: Buyya, R., Broberg, J, Goscinski, A., "Cloud Computing Principles and Paradigms", Wiley & Sons, 2011
Hwang, K., Fox, G., and Dongarra, J., "Distributed and Cloud Computing (From Parallel Processing to the Internet of Things)", Elsevier, 2011
Vincent W. S. (2018). Build websites with Python and Django. Ed: Independently published. ISBN-10: 1983172669. ISBN-13: 978-1983172663.
Authors:
Reference:
Year:
Title: --
Authors:
Reference:
Year:
Analytical Information Systems
The aim is to introduce the concepts and practical knowledge fundamental to the design and implementation of an analytical information system for an organization:
(LO1) Plan and manage the life cycle of a data warehouse project, logical and physical design;
(LO2) Identify requirements and data sources and design an appropriate dimensional model;
(LO3) Model an Analytical Information System;
(LO4) Design and implement a data extraction, transformation and loading process;
(LO5) Analyze data in a data warehousing system , have an understanding of Business Intelligence and its applicability; what are standard reports and performance indicators ( KPIs );
(CP1) SQL review: simple queries. Union of tables. Ordering. Grouping and aggregation.
(CP2) Data integration, introduction to ETL tools: data sources. Data integration using visualizations. Data integration versus data warehousing. ETL process. ETL tools.
warehouse design : OLAP operations in SQL. Multidimensional model. Modeling in UML. Typical OLAP operations on a data cube. Data warehouse schema types . Hierarchies and types of hierarchy. Measurements.
(CP4) The OLAP Cube and MDX queries - Data storage and OLAP cubes. OLAP server. OLAP cube definition. OLAP interface. Analysis queries. SQL versus MDX. MDX Concepts.
(CP5) Reporting tools and ( KPIs ) - Data warehousing architecture overview . OLAP tools: front-end and MDX queries . Reporting tools. Reports via SQL and MDX queries. Key performance indicators ( KPIs ). Visualization with dashboards .
Periodic assessment results from the following components:
- A midterm test (20% of the final grade) and another at the end of the semester (20% of the final grade);
- Group work (maximum 3 students) in which the group will develop an analytical information system with the writing of a report which involves 3 deliverables to be submitted along the semester(30% of the final grade, 10% each deliverable) and an oral presentation with a demonstration of the operation of the developed application and discussion (30% of the final grade).
Alternatively, students may choose to be assessed in a final exam (100% of the final grade)
Students who obtain a final grade above 9.5 are approved.
Title: Vaisman, A., & Zimányi, E. (2014). Data warehouse systems. Data-Centric Systems and Applications.
Secundária, Springer
Authors:
Reference:
Year:
Title: R. Kimball, M. Ross (2013) The Data Warehouse Toolkit - the definitive guide to dimensional modeling, 3rd Edition. John Wiley & Sons, USA
Doan, A., Halevy, A., & Ives, Z. (2012). Principles of data integration. Elsevier.
Authors:
Reference:
Year:
Big Data
At the end of the course students should be able to
OA1 Understand and identify the problems associated with the processing of large amounts of data and information
LO2 Understand the concepts and ecosystem of Big Data
LO3 Design and implement solutions for data storage in a distributed and fault tolerant environment
LO4 Extract, transform and load large amounts of information from unstructured data sources
LO5 Know how to manipulate and process non-relational databases
LO6 Understand and now how to apply distributed programming and computing models
LO7 Understand and now how to apply techniques for processing JSON structures and real time data streams
LO8 Develop creativity, technological innovation, critical thinking
LO9 Develop self-learning, peer review, teamwork, verbal and oral expression
S1 The concept of Big Data, applicable problems and its ecosystem
S2 Introduction to non-relational databases and MongoDB
S3 Computing architecture for Big Data: (1) redundant and fault tolerant and (2) distributed to support large volumes of data. Example of the Hadoop platform and its distributed file system
S4 The MapReduce programming model
S5 Designing databases in MongoDB
S6 Manipulation of JSON structures and real-time data
S7 The ETL - Extract, Transform and Load process aplied to unnormalized data sets and development of Big Data processing applications in Spark and MongoDB environments
Course with Periodic Assessment, not by Final Exam. Presence is required in 90% of all activities.. Assessment weights:
- Individual practical assignments, 80% of which are compulsory (25%)
- Lab project (in group of 2), with individual oral discussion (50%)
- 2 multiple response Mini-tests (25%)
A mark below 10 assigns the student to an exam in the normal and/or the appeal period (50% of the mark), with the completion and approval of the group project, or an individual project mandatory (50%).
Title: Advanced Analytics with Spark: Patterns for Learning from Data at Scale, Sandy Ryza et al., O'Reilly Media, 2017.
Practical Data Science with Hadoop and Spark: Designing and Building Effective Analytics at Scale, Ofer Mendelevitch, Casey Stella and Douglas Eadline, Addison-wesley, 2016.
NoSQL Database: New Era of Databases for Big data Analytics - Classification, Characteristics and Comparison, A B M Moniruzzaman, Syed Akhter Hossain, 2013 (https://arxiv.org/abs/1307.0191)
Kumar, V. Shindgikar, P. (2018). Modern Big Data Processing with Hadoop. Ed: Packt. ISBN-13: 978-1-78712-276-5
Big Data: Algorithms, Analytics, and Applications, Kuan-Ching Li et al., Chapman and Hall/CRC, 2015.
Authors:
Reference:
Year:
Title: Chang F., Dean J., Ghemawat S,, C. Hsieh W., Wallach D., Burrows M., Chandra T., Fikes A., and Gruber, R. (2006). Bigtable: a distributed storage system for structured data. In Proceedings of the 7th USENIX Symposium on Operating Systems Design and Implementation - Volume 7 (OSDI '06). USENIX Association, USA, 15.
Dean J. and Ghemawat S. (2004). MapReduce: simplified data processing on large clusters. In Proceedings of the 6th conference on Symposium on Operating Systems Design & Implementation - Volume 6 (OSDI'04). USENIX Association, USA, 10.
Ghemawat S., Howard G., and Leung, S. (2003). The Google file system. SIGOPS Oper. Syst. Rev. 37, 5 (December 2003), 29?43. DOI: https://doi.org/10.1145/1165389.945450
Artigos:
Learning Spark: Lightning-Fast Big Data Analysis, Holden Karau, A. Konwinski, P. Wendell and M. Zaharia, O'Reilly Media, 2015.
Marinescu, D. (2018). Cloud Computing: Theory and Practice. Ed: Morgan Kaufmann. ISBN-13: 978-0-12-812810-7
Mining of Massive Datasets, A. Rajaraman, J. Ullman, 2011, Cambridge University Press.
Authors:
Reference:
Year:
Applied Project in Artificial Intelligence I
At the end of the course, the student should be able to:
LO1: Apply co-creation methodologies in the development of innovative triple sustainable projects (with economic, social and environmental value) in organizations.
LO2: Create empathy with the user and his organization (define needs, obstacles, goals, opportunities, current and desired tasks), define the problem and raise the issues addressed by the project.
LO3: Conduct a systematic literature review and competitive landscape analysis (if applicable), related to the identified problem and the issues raised.
LO4: Identify the digital (including data collection), computational and other resources needed to address the problem.
LO5: Apply already consolidated knowledge of project planning, agile management and project development, within the framework of group work.
LO6: Participate in collaborative and co-creation dynamics and make written and oral presentations, in the context of group work.
S1. Co-creation methodologies based on Design Thinking and Design Sprint
C2. Sustainable Development Goals (SDGs) of the United Nations. Creation of value propositions
S3. Presentation of case studies and digital technologies project topics in artificial intelligence (product, service or process)
S4. Selecting the project topic and framing it in the organization
S5. Problem space: creating empathy with the user and his organization, defining the problem and its related issues, considering business requirements, customer and user needs, and technology challenges
S6. Application of a systematic literature review methodology and its critical analysis. Competition analysis (if applicable)
S7. Identification of digital resources (including data collection), computational, and other resources required for project development
S8. Application of agile project management methodologies, appropriate to the group work to be developed by the students artificial intelligence. Communication of results.
Course in periodic assessment, not contemplating final exam, given the adoption of the project-based teaching-learning method applied to real situations. Presentations, demonstrations and discussion will be carried out in groups.
Assessment weights:
R1 Report: Project Topic Definition: 5%.
R2 Report: Empathy with the User and the Organization and Definition of the Problem. Its presentation and group discussion: 40%
R3 Report: Systematic Literature Review and Project Development Planning. Its presentation and group discussion: 55%.
Title: ·
T. Brown / HarperCollins, 2009, ISBN-13: 978-0062856623, Change by Design: How Design Thinking Transforms Organizations and Inspires Innovation, 2009, ·, ·
A. Osterwalder, Y. Pigneur, P. Papadakos, G. Bernarda, T. Papadakos, A. Smith / John Wiley & Sons., Value proposition design, 2014, ·, ·
J. Knapp, J. Zeratsky, B. Kowitz, Sprint: How to Solve Big Problems and Test New Ideas in Just Five Days., 2016, ·, ·
M. Lewrick, P. Link, L. Leifer / Wiley, ISBN 9781119629191, The Design Thinking Toolbox, 2020, ·, ·
Authors:
Reference:
Year:
Title: ·
Ries, E. / capítulos 3 e 4, Penguin Group, The Lean Startup: How Today's Entrepreneurs Use Continuous Innovation to Create Radically Successful Businesses, 2017, ·, ·
Scrum Institute, The Kanban Framework 3rd Edition, 2020, ·, www.scrum-institute.org/contents/The_Kanban_Framework_by_International_Scrum_Institute.pdf acedido em 02/2023
Darrell Rigby, Sarah Elk, Steve Berez / Scrum Institute, The Scrum Framework 3rd Edition, Doing Agile Right: Transformation Without Chaos Hardcover, 2020, ·, www.scrum-institute.org/contents/The_Scrum_Framework_by_International_Scrum_Institute.pdf acedido em 02/2023
Jeff Sutherland, J.J. Sutherland, Scrum: The Art of Doing Twice the Work in Half the Time, 2014, ·, ·
Project Management Institute / 6th ed. Newton Square, PA: Project Management Institute, A guide to the project management body of knowledge (PMBOK guide), 2017, ·, ·
Gwaldis M., How to conduct a successful pilot: Fail fast, safe, and smart, 2019, ·, https://blog.shi.com/melissa-gwaldis/ acedido em 02/2023
Authors:
Reference:
Year:
Text Mining
"OA1: To describe the main concepts, steps and methods involved in the development of Text Mining processes
OA2: To atomize documents, create dictionaries and perform other pre-processing tasks in order to prepare text for classification tasks
OA3: To select appropriate techniques for specific text processing tasks
OA4: Create vector representations from texts
OA5: Explain the operation of algorithms for text classification, such as Naïve Bayes or nearest-neighbor
OA6: To apply a classifier to real cases
OA7: To group documents using k-means algorithm"
Introduction
CP1: Importance of large quantities of text, challenges and current methods
CP2: Unstructured vs. (semi-)structured information
CP3: Obtaining and filtering information, information extraction and Data Mining
Document Representation
CP4: Document pre-processing
CP5: Feature extraction: terms as features
CP6: Term weighting schemes
CP7: Vector space models
CP8: Similarity measures
Text Classification
CP9: Introduction to statistical machine learning
CP10: Evaluation
CP11: Supervised learning
CP12: Naïve Bayes
CP13: Nearest Neighbor
CP14: Sentiment analysis
CP15: Text Mining Resources
Clustering
CP16: k-means algorithm
This course can be concluded either by a periodic evaluation or by exam. The periodic evaluation consists of two components: TRABALHO (1 assignment, 40%) and MINI-TESTS (3 mini-tests, each worth 20%). The minimum score of each one of the components is 8 (in 20). In case of failure, or if the student chooses to be evaluated by an exam, the exam corresponds to 100% of the grade.
BibliographyTitle: - Dan Jurafsky and James H. Martin (Sep 2021). Speech and Language Processing (3rd ed. draft). https://web.stanford.edu/~jurafsky/slp3/
- Gabe Ignatow, Rada F. Mihalcea (2017). An Introduction to Text Mining: Research Design, Data Collection, and Analysis 1st Edition (2017). SAGE Publications
- Charu C. Aggarwal (2018). Machine Learning for Text. https://doi.org/10.1007/978-3-319-73531- 3
Authors:
Reference:
Year:
Title: - Atefeh Farzindar and Diana Inkpen (2017). Natural Language Processing for Social Media, Second Edition. Synthesis Lectures on Human Language Technologies. Morgan & Claypool.
Authors:
Reference:
Year:
Advanced Search Algorithms
At the end of this CU, the student should be able to:
LO1 Identify classes of problems solvable with advanced search algorithms
LO2 Master and apply the main types of advanced search algorithms
LO3 Master and apply heuristics as a solution, according to the type of problem to be solved
LO4 Identify and evaluate different strategies to be applied, looking at the complexity and nature of the problem
S1 HEURISTIC AND PROBLEM REPRESENTATION
a Types of problems for heuristics usage
b Search space and problem representation
c Optimization, satisfaction, and semi-optimization tasks
d Systematic search and the divide pruning paradigm
e State space representation
f Problem-reduction representation and graphs and/or
S2 BASIC HEURISTIC RESEARCH STRATEGIES
a Local search
b Uninformed systematic search
c Informed systematic search
d Specialized best first strategies
e Hybrid Strategies
S3 ADVANCED HEURISTIC RESEARCH STRATEGIES
a Memory-limited Strategies
b Time-limited strategies
S4 STRATEGIES WITH GENETIC ALGORITHMS
a The call of evolution
b Biological terminology
c State space search
d Elements of genetic algorithms (evaluation functions and genetic operators)
e A simple genetic algorithm
f Genetic algorithms versus traditional search methods
Periodic Evaluation:
- Group Project up to 3 students (40%)
- Individual Test (60%)
Final evaluation:
- Individual Exam (100%)
Students who fail the continuous assessment have two exam periods (2nd and Special).
The project grade is not considered for students who choose to take the exam.
Whoever chooses to take the continuous assessment will have to carry out the two components of it.
Title: An Introduction to Genetic Algorithms, Mitchell M. 1999 MIT Press
Heuristics, intelligent search strategies for computer problem-solving: Pearl J. 1984 Addison-Wesley
Authors:
Reference:
Year:
Title: Artificial Intelligence: A Modern Approach, 3rd edition: Stuart Russel and Peter Norvig 2010 Pearson / Prentice Hall
Authors:
Reference:
Year:
Applied Project in Artificial Intelligence II
LO1: Correct the user and/or organization problem identified in the Applied Project I course of the 1st semester, developing, in an iterative way, an integrated project with all its components, including requirements gathering, solution prototyping (lo-fi, hi-fi, MVP), and evaluation and field deployment of the innovative solution, regarding product, process or service (PPS).
LO2: Produce design documentation of the PPS innovation solution, including, where applicable, architecture, hardware and software configuration, installation, operation and usage manuals.
LO3: Produce solutions with the potential to be triple sustainable in the field, taking into account the applicable legal framework.
LO4: Produce audiovisual content on the achieved results, to be exploited in several communication channels: social networks, landing page web, presentation to relevant stakeholders, demonstration workshop.
S1. Solution space: ideation of the best technological solution relative to the project, development of user requirements, storyboarding, user/costumer journey, iterative prototyping cycles (low fidelity - lo-fi, high fidelity - hi-fi, minimum viable product - MVP), heuristic evaluation of the solution with experts and evaluation with end users.
S2. Production of solution design documentation, including, where applicable, architecture, technical specifications, hardware and software configuration, installation, operation and use manuals.
S3. Experimental deployment of the solution with the potential to be triple sustainable (with economic, social and environmental value creation), safeguarding the applicable legal framework.
S4. Audiovisual communication on the Web and social networks. Communication in public and its structure. Presentation to relevant actors.
S5. Demonstration in workshop with relevant actors in the field of Artificial Intelligence.
UC in periodic assessment, not contemplating final exam, given the adoption of the project-based teaching method applied to real situations. Presentations, demonstrations and discussion are carried out in groups.
Evaluation weights:
R1 Solution Ideation Report, with Storyboard, User Journey, User Requirements, Technical Specifications and its audiovisual presentation: 20%.
R2 Solution Prototyping: Lo-fi and Hi-fi Prototypes and Minimum Viable Prototype - MVP (on GitHub), its Demonstration and Evaluation Report: 40%
R3 Solution Design Report with the following elements (if applicable): Architecture (UML Package Diagram, UML Component Diagram), Hardware and Software Configuration, Installation Manual (UML Deployment Diagram, Configuration Tutorial), Operation Manual, User Manual: 20%
R4 Audio-visual presentation of the solution and its demonstration in a Workshop: 20%.
Title: T. Brown, HarperCollins, 2009, ISBN-13: 978-0062856623, Change by Design: How Design Thinking Transforms Organizations and Inspires Innovation, 2009, ·, ·
M. Lewrick, P. Link, L. Leifer / Wiley, ISBN 9781119629191, The Design Thinking Toolbox, 2020, ·, ·
J. Knapp, J. Zeratsky, B. Kowitz / Bantam Press., Sprint: How to Solve Big Problems and Test New Ideas in Just Five Days., 2016, ·, ·
Authors:
Reference:
Year:
Title: ·
E. Ries, The Lean Startup: How Today's Entrepreneurs Use Continuous Innovation to Create Radically Successful Businesses, Capítulos 3 e 4, Penguin Group, 2017, ·, ·
Scrum Institute, The Kanban Framework 3rd Edition, 2020, ·, www.scrum-institute.org/contents/The_Kanban_Framework_by_International_Scrum_Institute.pdf acedido em 02/2023
Darrell Rigby, Sarah Elk, Steve Berez / The Scrum Framework 3rd Edition, Doing Agile Right: Transformation Without Chaos HardcoverScrum Institute, 2020, ·, www.scrum-institute.org/contents/The_Scrum_Framework_by_International_Scrum_Institute.pdf
Jeff Sutherland, J.J. Sutherland, Scrum: The Art of Doing Twice the Work in Half the Time, 2014, ·, ·
Project Management Institute. / 6th ed. Newton Square, PA: Project Management Institute, A guide to the project management body of knowledge (PMBOK guide)., 2017, ·, ·
M. Gwaldis, How to conduct a successful pilot: Fail fast, safe, and smart / https://blog.shi.com/melissa-gwaldis/ acedido em 02/2023, 2019, ·, ·
Authors:
Reference:
Year:
Technology, Economy and Society
The student who successfully completes this UC will be able to:
OA1. Identify the main contemporary issues and debates;
OA2. Analyze current issues and debates in a reasoned manner;
OA3. Identify the implications of technological change and digitalization in economic, social, cultural and environmental terms;
OA4. Understand the role and the importance of technology in the challenges of contemporary societies;
OA5. Explore the boundaries between technological knowledge and social science knowledge;
OA6. Develop forms of interdisciplinary learning and critical thinking.
S1. Debates XXI: technological change and contemporary societal challenges.
S2. Digital transition: meaning and implications.
S3. Technology, social change and inequalities.
S4. Environment and transition towards to sustainability.
S5. Globalization, financialisation and development.
S6. Capitalism and democracy.
S7. Migrations and multiculturality.
The periodic assessment process comprises the following elements:
1. Preparation and presentation (class) of a group work on technological change and society (40%).
2. Test (60%).
The final assessment corresponds to 1st and 2nd phase exams (100% of the grade).
Title: Pires, R. P.; Pereira, C.; Azevedo, J.; Vidigal, I., & Veiga, C. M. (2020). A emigração portuguesa no século XXI.?Sociologia, Problemas e Práticas, (94), 9-38
Marques, P., & Salavisa, I. (2017). Young people and dualization in Europe: a fuzzy set analysis.?Socio-Economic Review,?15(1), 135-160
Figay, N.; Silva, C.; Ghodous, P; Jardim-Gonçalves, R. (2015). Resolving interoperability in concurrent engineering, in Concurrent Engineering in the 21st Century: Foundations, Developments and Challenges, Springer International Publishing
Bento, N., Wilson, C., Anadon, L.D. (2018), ?Time to get ready: Conceptualizing the temporal and spatial dynamics of formative phases for energy technologies,? Energy Policy 119: 282-293
Barradas, R., & Lagoa, S. (2017). Financialization and Portuguese real investment: A supportive or disruptive relationship?.?Journal of Post Keynesian Economics,?40(3), 413-439
Authors:
Reference:
Year:
Title: Yearley, S. (2014).?Science, Technology, and Social Change (Routledge Revivals). Routledge
Wilson, C., Grubler, A., Bento, N., Healey, S., De Stercke, S., & Zimm, C. (2020). Granular technologies to accelerate decarbonization.?Science,?368(6486), 36-39
Silva, P. A., & Cadeiras, P. (2019). From Paris to Lisbon: The Ever-Changing European Social Policy Landscape. In?The Future of Pension Plans in the EU Internal Market?(pp. 255-281). Springer, Cham
Silva, J., Ferreira, J. C., & Gonçalves, F. (2019, September). The ??aftermath??of Industry 4.0 in Small and Medium Enterprises. In?IFIP Conference on Human-Computer Interaction?(pp. 26-33). Springer, Cham
Rodrigues, M. D. L., & Silva, P. A. (2016). A constituição e as políticas públicas em Portugal.?Sociologia, Problemas e Práticas, (ESP1), 13-22
Rego, R., Alves, P. M., Naumann, R., & Silva, J. (2014). A typology of trade union websites with evidence from Portugal and Britain.?European Journal of Industrial Relations,?20(2), 185-195
Ratto, M. (2011). Critical making: Conceptual and material studies in technology and social life.?The information society,?27(4), 252-260
Pires, R. P., Machado, F. L., Peixoto, J., & Vaz, M. J. (2010). Portugal: Atlas das migrações internacionais.?Lisboa: Tinta da China
Pedro, M. D. L. R. E., & Silva, A. E. (2012).?Políticas públicas em Portugal. Leya
Nascimento, S., Pólvora, A., Paio, A., Oliveira, S., Rato, V., Oliveira, M. J., ... & Sousa, J. P. (2016). Sustainable technologies and transdisciplinary futures: from collaborative design to digital fabrication.?Science as Culture,?25(4), 520-537
Monteiro, V., Afonso, J. A., Ferreira, J. C., & Afonso, J. L. (2019). Vehicle electrification: New challenges and opportunities for smart grids.?Energies,?12(1), 118.
Matthewman, S. (2011).?Technology and social theory. Macmillan International Higher Education
Matos, F. (2020).?Knowledge, People, and Digital Transformation: Approaches for a Sustainable Future. Springer Nature
Luís, S., Pinho, L., Lima, M. L., Roseta-Palma, C., Martins, F. C., & Betâmio de Almeida, A. (2016). Is it all about awareness? The normalization of coastal risk.?Journal of Risk Research,?19(6), 810-826
Leach, M., Scoones, I., & Stirling, A. (2010).?Dynamic sustainabilities: technology, environment, social justice. Routledge
Lagoa, S., Leao, E., Mamede, R. P., & Barradas, R. (2014).?Financialisation and the financial and economic crises: The case of Portugal?(No. fstudy24). Financialisation, Economy, Society & Sustainable Development (FESSUD) Project
Grubler, A., Wilson, C., Bento, N., Boza-Kiss, B., Krey, V., McCollum, D. L., ... & Valin, H. (2018). A low energy demand scenario for meeting the 1.5 C target and sustainable development goals without negative emission technologies.?Nature energy,?3(6), 515-527
Jörgens, H., & Solorio, I. (2019). Contested Energy Transition? Europeanization and Authority Turns in EU Renewable Energy Policy
Jörgens, H. (2018). Políticas para um desenvolvimento sustentável: sucessos passados e desafios para o futuro
Jörgens, H., Goritz, A., & Kolleck, N. (2018). Vantagens e desafios da análise de dados do Twitter: O caso das negociações multilaterais sobre as mudanças climáticas
Horta, P., Lagoa, S., & Martins, L. (2016). Unveiling investor-induced channels of financial contagion in the 2008 financial crisis using copulas.?Quantitative Finance,?16(4), 625-637
Frois, C. (2013).?Peripheral vision: Politics, technology, and surveillance?(Vol. 22). Berghahn Books
Facer, K. (2011).?Learning futures: Education, technology and social change. Taylor & Francis
Berbel, J., Borrego-Marin, M., Exposito, A., Giannoccaro, G., Montilla-Lopez, N. M., & Roseta-Palma, C. (2019). Analysis of irrigation water tariffs and taxes in Europe.?Water Policy,?21(4), 806-825
Bento, N. (2010). Dynamic competition between plug-in hybrid and hydrogen fuel cell vehicles for personal transportation.?International journal of hydrogen energy,?35(20), 11271-11283
Bento, N., & Fontes, M. (2015). Spatial diffusion and the formation of a technological innovation system in the receiving country: The case of wind energy in Portugal.?Environmental Innovation and Societal Transitions,?15, 158-179
Bento, N. (2016). Calling for change? Innovation, diffusion, and the energy impacts of global mobile telephony.?Energy Research & Social Science,?21, 84-100.
Bento, N., & Fontes, M. (2019). Emergence of floating offshore wind energy: Technology and industry.?Renewable and Sustainable Energy Reviews,?99, 66-82
Bento, N., Gianfrate, G., & Thoni, M. H. (2019). Crowdfunding for sustainability ventures.?Journal of Cleaner Production,?237, 117751
Barak, M. (2017). Science teacher education in the twenty-first century: A pedagogical framework for technology-integrated social constructivism.?Research in Science Education,?47(2), 283-303.
Authors:
Reference:
Year:
Recommended optative
Objectives
Accreditations
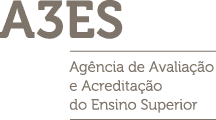